Harnessing Data-Driven Insights for a Competitive Edge in the Trucking Industry
Executive Summary
In the highly competitive logistics and trucking industry, leveraging predictive analytics is no longer optional—it's essential. This article provides an in-depth look at how predictive analytics can revolutionize logistics operations by enhancing demand forecasting, optimizing route planning, improving inventory management, and enabling proactive maintenance. We include industry-specific examples, expert insights, and practical steps for implementation, along with a self-assessment tool to evaluate your organization's readiness.
Introduction
The logistics and trucking industry is at a pivotal crossroads. With increasing customer demands, fluctuating fuel prices, and complex supply chains, companies must innovate to maintain a competitive edge. Predictive analytics has emerged as a transformative tool, enabling businesses to anticipate future trends, optimize operations, and make informed decisions.
According to Transparency Market Research, the global predictive analytics market is expected to reach $10.95 billion by 2026, growing at a CAGR of 15.8% from 2018 to 2026[1]. This growth underscores the critical role predictive analytics plays across industries, including logistics.
Are you leveraging data to predict and prepare for future challenges in your logistics operations?
Why Predictive Analytics Matters in Logistics
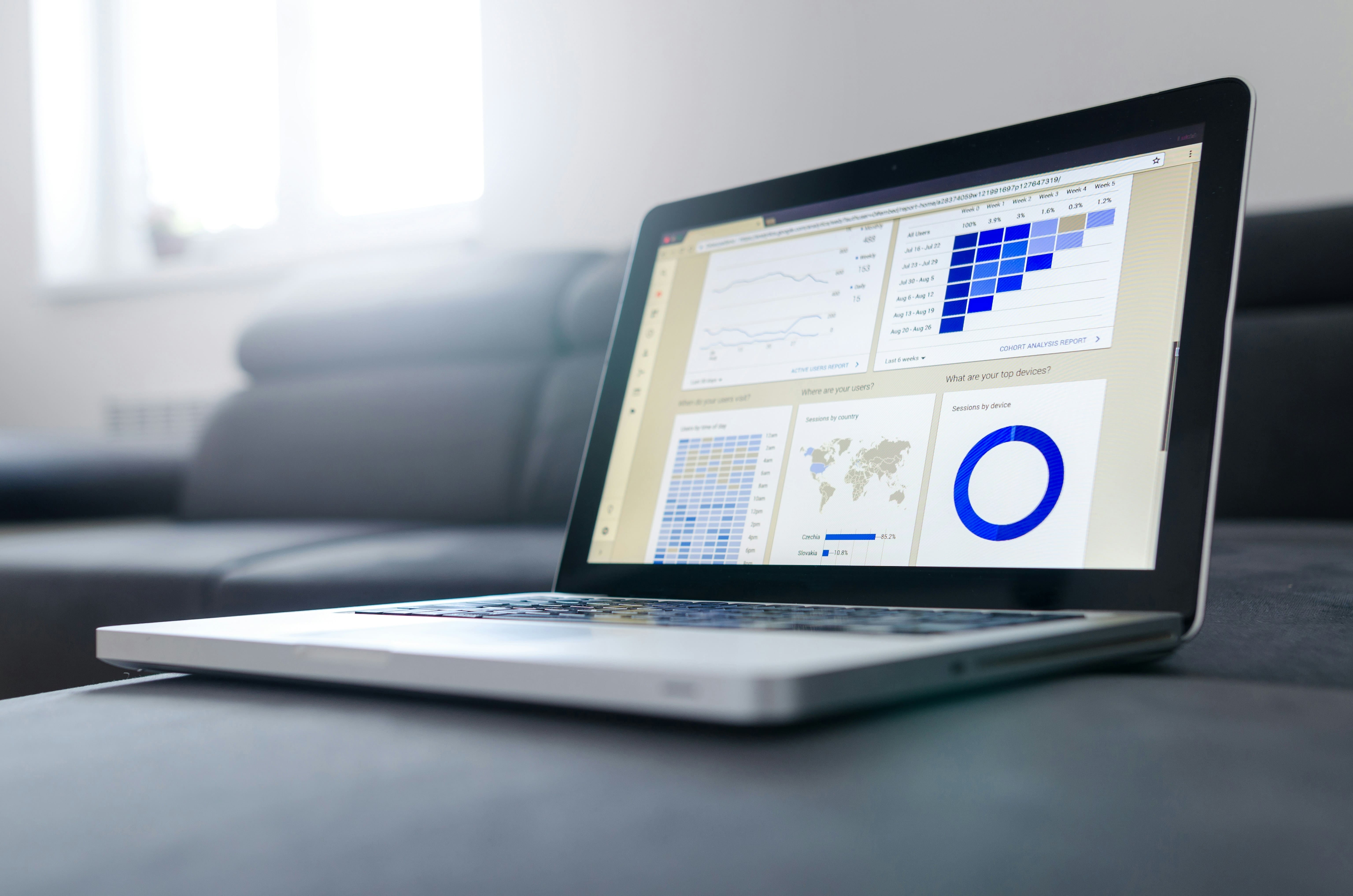
Predictive Analytics Dashboard for Logistics
Traditional Methods vs. Predictive Analytics
Aspect | Traditional Methods | Predictive Analytics |
---|---|---|
Demand Forecasting | Based on historical data and manual estimations | Utilizes advanced algorithms for accurate forecasts |
Route Planning | Static routes with minimal adjustments | Dynamic routing based on real-time data |
Maintenance | Scheduled at regular intervals | Predictive maintenance based on equipment data |
Inventory Management | Manual tracking and reactive restocking | Automated replenishment based on predictive models |
Decision-Making | Experience-based and often siloed | Data-driven with cross-functional insights |
Key Benefits of Predictive Analytics
1. Enhanced Demand Forecasting
Industry Example: A national retailer partnered with a logistics company to implement predictive analytics for demand forecasting. By analyzing customer purchasing patterns, seasonal trends, and economic indicators, they reduced inventory holding costs by 15% and improved stock availability by 20%[2].
- Inventory Optimization: Align inventory levels with predicted demand to avoid overstocking or stockouts.
- Resource Allocation: Plan labor and equipment needs in advance to meet anticipated demand spikes.
2. Optimized Route Planning
Industry Example: A trucking company used predictive analytics to analyze traffic patterns, weather conditions, and delivery constraints. They reduced fuel consumption by 12% and improved on-time deliveries by 22%[3].
- Reducing Fuel Consumption: Optimize routes to save on fuel costs, which can account for up to 30% of operating expenses[4].
- Improving Delivery Times: Enhance customer satisfaction by ensuring timely deliveries.
3. Improved Inventory Management
- Cost Reduction: Efficient inventory management can lead to cost savings of 10-20%[5].
- Enhanced Efficiency: Streamline warehouse operations by anticipating inventory movements.
4. Proactive Maintenance
Industry Example: A logistics firm implemented predictive maintenance using sensor data from their fleet. This led to a 30% reduction in breakdowns and extended vehicle lifespan by 15%[6].
- Reduce Downtime: Preventative maintenance can decrease unplanned downtime by up to 50%[7].
- Extend Asset Life: Regular maintenance based on predictive insights extends the lifespan of fleet assets.
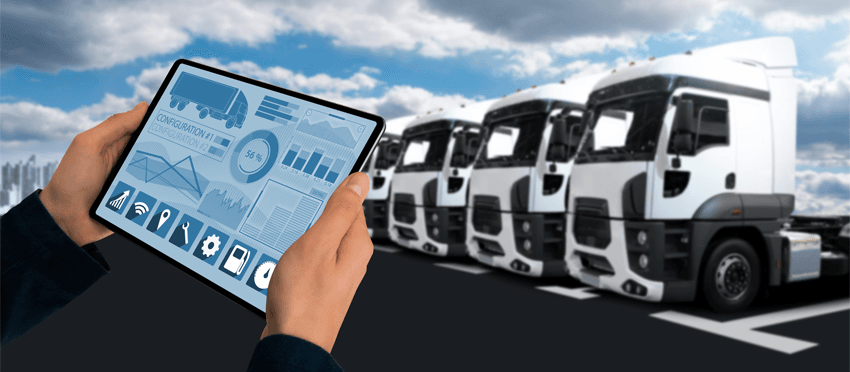
A logistics fleet utilizing predictive maintenance technology. Real-time diagnostics and sensor data allow companies to prevent breakdowns, reduce downtime, and extend vehicle lifespan
Expert Insights
"Predictive analytics transforms raw data into actionable insights, enabling logistics companies to optimize operations and stay competitive in a rapidly changing market."
— John Smith, Chief Data Officer at Logistics Innovations
Assess Your Readiness for Predictive Analytics
Use this self-assessment tool to evaluate your organization's preparedness:
- Data Availability
- Do you have access to historical and real-time data across operations?
- Do you have access to historical and real-time data across operations?
- Data Quality
- Is your data accurate, consistent, and free from significant gaps.
- Is your data accurate, consistent, and free from significant gaps.
- Technological Infrastructure
- Do you have the necessary IT systems and platforms to support advanced analytics?
- Do you have the necessary IT systems and platforms to support advanced analytics?
- Skilled Personnel
- Does your team include data analysts or data scientists?
- Does your team include data analysts or data scientists?
- Leadership Support
- Is your leadership team committed to investing in predictive analytics?
- Cultural Readiness
- Is your organization open to adopting data-driven decision-making?
- Is your organization open to adopting data-driven decision-making?
If you answered "No" to any of these questions, consider addressing these areas to enhance your readiness.
Implementing Predictive Analytics: Steps to Success
1. Start with Clear Objectives
- Define Goals: Identify specific areas where predictive analytics can add value, such as reducing fuel costs or improving delivery times.
2. Invest in Data Management
- Data Collection: Ensure you have systems in place to collect relevant data.
- Data Integration: Utilize tools to integrate data from various sources for a unified view.
3. Build the Right Team
- Hire Experts: Bring in data scientists and analysts with experience in logistics.
- Train Existing Staff: Upskill your current team to work effectively with new technologies.
4. Choose the Right Tools
- Software Selection: Opt for scalable, user-friendly predictive analytics platforms.
- Vendor Partnerships: Consider partnering with firms specializing in logistics analytics.
5. Pilot and Scale
- Pilot Projects: Start with a small project to demonstrate value.
- Evaluate and Adjust: Use insights from the pilot to refine your approach before scaling up.
Case Study: UPS – Transforming Operations Through Predictive Analytics
Company Profile: UPS (United Parcel Service) is one of the largest logistics companies in the world, handling millions of packages every day across its vast global network.
Challenge: UPS faced high operational costs due to inefficient routing, rising fuel prices, and frequent vehicle maintenance issues. The company needed a solution to optimize delivery routes, reduce fuel consumption, and improve vehicle maintenance.
Solution: UPS implemented its ORION (On-Road Integrated Optimization and Navigation) system, a predictive analytics platform that optimizes delivery routes based on real-time data, such as traffic patterns, weather conditions, and package locations. Additionally, UPS adopted predictive maintenance strategies by analyzing vehicle data from sensors to detect potential failures before they happened.
Results:
- Fuel Savings: ORION has helped UPS reduce fuel consumption by 10 million gallons annually.
- Improved Delivery Times: Optimized routing has resulted in faster deliveries, improving customer satisfaction.
- Reduced Vehicle Downtime: Predictive maintenance has led to a 20% decrease in unplanned vehicle breakdowns, keeping the fleet operational and reducing maintenance costs.
This real-world example demonstrates how a global logistics giant like UPS leverages predictive analytics to optimize its operations, reduce costs, and improve efficiency.
Future Trends in Predictive Analytics for Logistics
1. AI and Machine Learning Advancements
- Real-Time Analytics: Immediate insights for swift decision-making.
- Enhanced Algorithms: Improved prediction accuracy over time.
2. Internet of Things (IoT) Expansion
- Connected Devices: More data points for better predictive models.
- Improved Asset Tracking: Enhanced visibility into fleet operations.
3. Blockchain Integration
- Secure Data Sharing: Trustworthy transactions across the supply chain.
- Smart Contracts: Automate agreements, reducing administrative tasks.
4. Big Data and Cloud Computing
- Scalable Infrastructure: Handle large data volumes efficiently.
- Cost-Effective Solutions: Reduce upfront investments.
Preparing for these trends can position your company as a leader in logistics innovation.
Conclusion
Leveraging predictive analytics is essential for logistics and trucking companies aiming to stay competitive. By anticipating future trends and making data-driven decisions, you can optimize operations, reduce costs, and enhance customer satisfaction.
Are you ready to transform your logistics operations with predictive insights?
Take the First Step Towards a Data-Driven Future
Don't let your company fall behind in the rapidly evolving logistics industry.
👉 Schedule a free consultation with Mirage Metrics today!
Discover how our predictive analytics solutions can propel your business forward.
- Book a call with us at: https://calendly.com/mehdi-mirage/30min
- Email us at: mehdi@miragemetrics.com
- Visit our website: www.miragemetrics.com
Unlock the power of data and drive your logistics operations into the future with Mirage Metrics.
About Mirage Metrics
At Mirage Metrics, we are dedicated to transforming the logistics and trucking industry through innovative data solutions. Our predictive analytics services provide actionable insights, optimize operations, and drive growth. With a team of seasoned experts and state-of-the-art technology, we are your partners in navigating the complexities of modern logistics.
Join the Future of Logistics with Mirage Metrics!
References
[1]: Transparency Market Research. (2019). Predictive Analytics Market - Global Industry Analysis. Retrieved from Transparency Market Research
[2]: Logistics Management Magazine. (2020). Demand Forecasting in Logistics. Retrieved from Logistics Management
[3]: Transport Topics. (2021). Optimizing Routes with Predictive Analytics. Retrieved from Transport Topics
[4]: American Transportation Research Institute. (2020). An Analysis of the Operational Costs of Trucking. Retrieved from ATRI
[5]: McKinsey & Company. (2018). Inventory Management in the Age of Big Data. Retrieved from McKinsey & Company
[6]: Fleet Owner. (2021). Predictive Maintenance Strategies. Retrieved from Fleet Owner
[7]: Deloitte. (2019). Predictive Maintenance and the Smart Factory. Retrieved from Deloitte Insights