Unlocking Operational Excellence through Artificial Intelligence
Executive Summary
The trucking industry is undergoing a seismic shift, driven by advancements in Artificial Intelligence (AI). This definitive guide delves deep into how AI technologies—such as machine learning, deep learning, and natural language processing—are revolutionizing route optimization, predictive maintenance, autonomous driving, and more. By providing in-depth technical details, trucking-specific data and benchmarks, and insights from industry veterans, this article serves as an essential resource for decision-makers aiming to lead their organizations into the future of trucking.
Introduction
Artificial Intelligence is not just a technological buzzword; it's a transformative force reshaping the trucking industry. With the potential to significantly enhance efficiency, safety, and profitability, AI is key to unlocking operational excellence. This guide provides an in-depth exploration of AI's impact, offering actionable insights for decision-makers poised to lead the next generation of trucking.
Understanding AI Technologies in Trucking
Machine Learning
Machine Learning (ML) is a subset of AI that enables systems to learn from data and improve over time without explicit programming.
- Use Cases in Trucking: Predictive maintenance, demand forecasting, fuel optimization, driver behavior analysis.
- How It Works: Algorithms identify patterns in historical and real-time data to make predictions or decisions. For example, ML models can analyze vast amounts of engine sensor data to predict potential failures before they occur.
Deep Learning
Deep Learning is a type of ML involving neural networks with multiple layers, mimicking the structure of the human brain.
- Use Cases: Image and video recognition for autonomous driving, speech recognition for driver assistance systems.
- How It Works: Processes large amounts of data through multiple layers of artificial neurons to recognize complex patterns and make decisions.
Natural Language Processing
Natural Language Processing (NLP) allows computers to understand, interpret, and generate human language.
- Use Cases: Voice-controlled systems in trucks, chatbots for customer service, analyzing driver logs.
- How It Works: Combines computational linguistics with ML to process and analyze large amounts of language data.
AI Applications in Trucking Operations
Route Optimization
How AI Algorithms Work
AI-powered route optimization involves several complex steps:
- Data Input: Incorporates real-time traffic data, weather conditions, vehicle load, driver hours of service (HOS) compliance, delivery time windows, and road restrictions (e.g., weight limits, low bridges).
- Processing: Advanced algorithms such as genetic algorithms, reinforcement learning, or mixed-integer linear programming evaluate millions of potential route combinations to identify the most efficient paths.
- Output: Provides optimized routes that minimize total distance, fuel consumption, and delivery times while complying with regulations and customer requirements.
Benefits
- Efficiency Gains: Studies show that AI-driven route optimization can reduce total miles driven by up to 10-15%[1].
- Cost Savings: Reduction in fuel costs and vehicle wear and tear.
- Improved Customer Satisfaction: More accurate delivery ETAs and higher on-time delivery rates.
Trucking-Specific Data and Benchmarks
- Average Fuel Savings: Companies implementing AI route optimization report average fuel savings of 5-10%.
- Reduction in Empty Miles: AI can reduce empty miles (deadheading) by matching backhauls, leading to a 30% reduction in some cases.
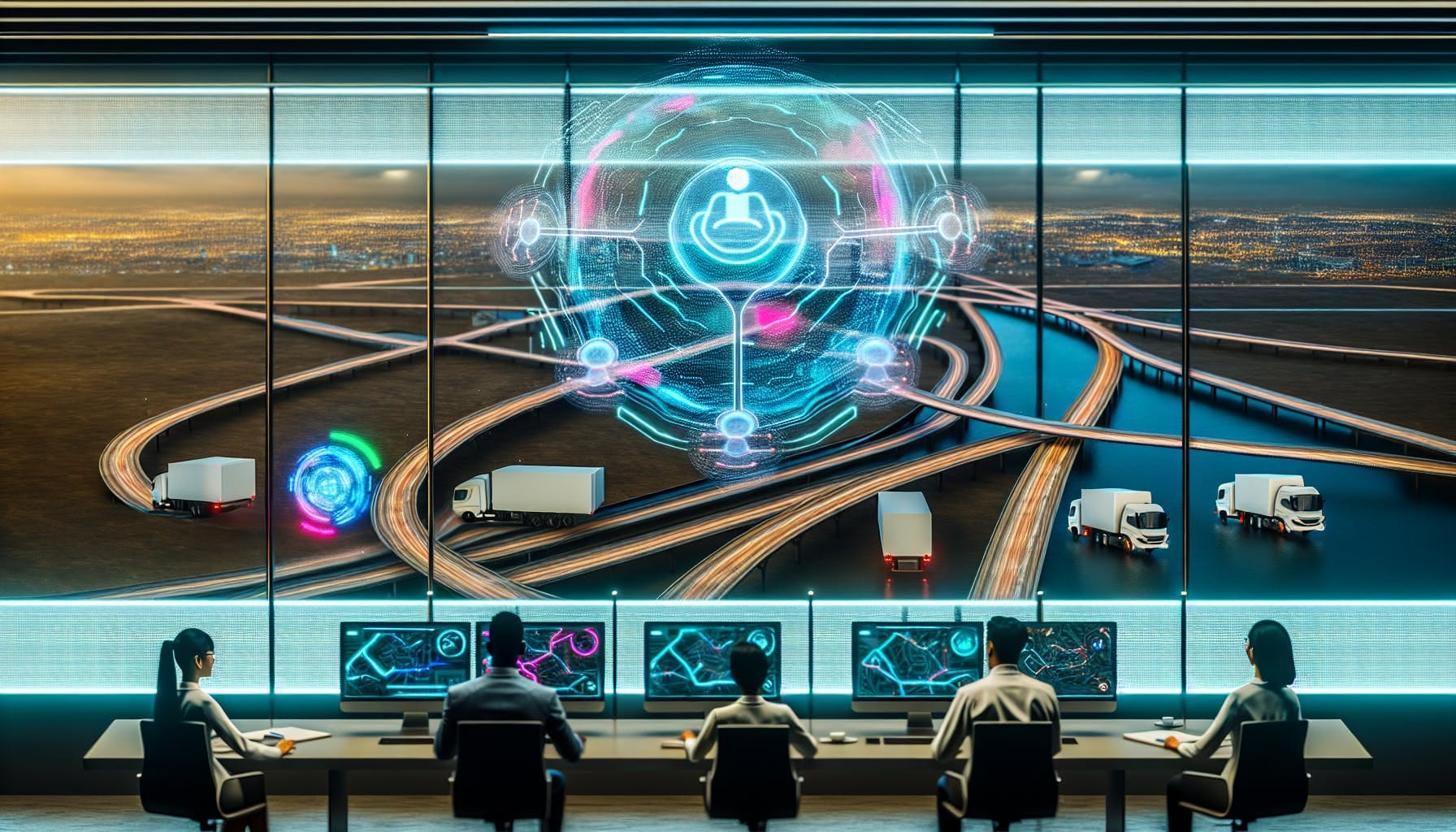
AI-powered dashboard optimizing truck routes in real-time to reduce fuel consumption and improve delivery times.
Predictive Maintenance
How AI Algorithms Work
- Data Collection: Sensors on vehicles collect data on engine temperature, oil pressure, vibration patterns, brake wear, and more.
- Data Processing: AI models, often using time-series analysis and anomaly detection algorithms, analyze this data to detect patterns indicative of potential failures.
- Predictive Insights: The system predicts the Remaining Useful Life (RUL) of components and schedules maintenance before a failure occurs.
Benefits
- Reduced Downtime: By preventing unexpected breakdowns, fleet availability is increased.
- Cost Savings: Maintenance costs can be reduced by up to 20% due to timely interventions.
- Safety Improvements: Ensures vehicles are in optimal condition, reducing the risk of accidents.
Trucking-Specific Data and Benchmarks
- Average Reduction in Breakdown Incidents: Fleets using predictive maintenance see a 30% reduction in breakdown incidents[2].
- Increase in Vehicle Lifespan: Proper maintenance can extend the lifespan of trucks by 15%.
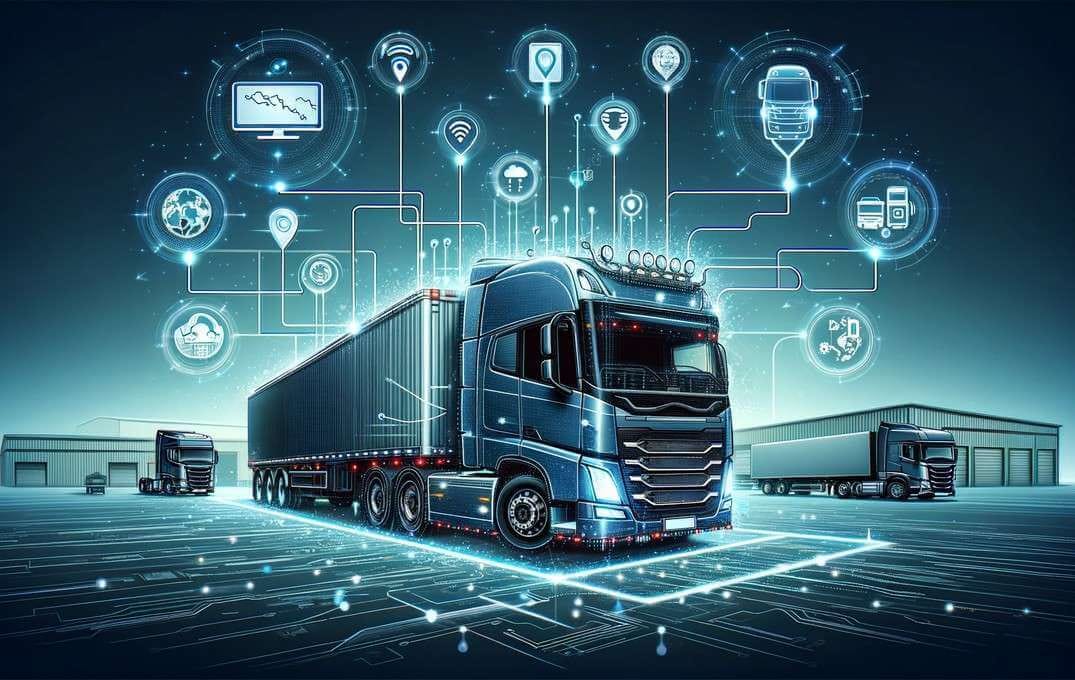
AI-driven predictive maintenance reduces vehicle breakdowns by monitoring real-time sensor data.
Autonomous Driving
How AI Algorithms Work
- Perception: AI uses sensor data (LiDAR, radar, cameras) to perceive the environment.
- Decision-Making: Deep learning models process this data to understand road conditions, traffic signals, obstacles, and make driving decisions.
- Control Execution: AI controls acceleration, braking, and steering to navigate the truck safely.
Benefits
- Safety Enhancements: Autonomous systems can react faster than humans, reducing the risk of accidents caused by human error.
- Operational Efficiency: Autonomous trucks can operate for longer hours without fatigue, increasing asset utilization.
- Fuel Efficiency: AI can optimize driving patterns for better fuel economy.
Trucking-Specific Data and Benchmarks
- Projected Safety Improvement: Autonomous trucks could reduce accidents by up to 40%[3].
- Regulatory Hurdles: Currently, regulatory approval is required for autonomous trucks to operate without a driver in most jurisdictions.
Demand Forecasting and Load Optimization
How AI Algorithms Work
- Data Sources: Historical shipment data, market trends, economic indicators, weather patterns, and even social media sentiment.
- Processing: Machine learning models like regression analysis, neural networks, or time-series forecasting predict future demand.
- Load Optimization: AI algorithms optimize load planning to maximize trailer space and reduce empty miles.
Benefits
- Inventory Management: Helps shippers and carriers plan for fluctuations in demand.
- Revenue Optimization: Better matching of loads with available capacity increases revenue per mile.
- Reduced Environmental Impact: Fewer empty runs mean lower carbon emissions.
Trucking-Specific Data and Benchmarks
- Increase in Load Factor: AI can improve load factors by 5-10%.
- Reduction in Empty Miles: Companies like Convoy report up to a 45% reduction in empty miles using AI-powered freight matching [4].
Future Scenarios: The AI-Integrated Trucking Operation
5-Year Outlook
- Semi-Autonomous Features: Widespread adoption of Level 2 and Level 3 autonomous features like adaptive cruise control and lane-keeping assistance.
- AI-Driven Decision Support: Dispatchers and fleet managers increasingly rely on AI for decision-making support.
- Data-Driven Operations: Most large fleets use AI for predictive maintenance and route optimization.
10-Year Vision
- Advanced Autonomous Trucks: Level 4 autonomy becomes commercially viable on highways, with drivers taking over in complex environments.
- Connected Ecosystems: Integration of AI systems across the supply chain for real-time coordination.
- Regulatory Evolution: Governments establish clearer frameworks for autonomous vehicle operation.
20-Year Paradigm Shift
- Fully Autonomous Fleets: Level 5 autonomous trucks operate without human intervention.
- AI-Orchestrated Logistics: AI systems manage end-to-end logistics, including warehousing and last-mile delivery.
- New Business Models: Emergence of AI-managed freight networks, possibly reducing the role of traditional carriers.
Ethical and Societal Implications
Workforce Impact
- Job Displacement: Estimates suggest that automation could affect up to 2.3 million trucking jobs in the U.S. (Source: American Trucking Associations).
- Transition Strategies:
- Reskilling Programs: Training drivers for new roles in fleet management, maintenance, or technology oversight.
- Education Partnerships: Collaborate with vocational schools and community colleges to develop relevant programs.
Ethical Decision-Making
- Autonomous Vehicle Ethics:
- Algorithmic Decisions: Programming how an autonomous vehicle should behave in unavoidable accident scenarios raises ethical questions.
- Accountability: Determining liability in accidents involving AI-driven vehicles is complex.
Effects on Small Operators
- Competitive Disadvantage: Smaller fleets may lack the capital to invest in AI technologies, widening the gap with larger carriers.
- Mitigation Strategies:
- Technology Partnerships: Joining cooperative platforms or networks that provide access to AI tools.
- Government Support: Advocating for subsidies or grants to assist small businesses in adopting AI.
In-Depth Case Studies
Case Study 1: UPS and ORION
Overview: UPS developed the On-Road Integrated Optimization and Navigation (ORION) system to optimize delivery routes.
Challenges:
- Managing complex delivery routes with multiple constraints.
- Controlling rising fuel costs and reducing environmental impact.
AI Implementation:
- Algorithms Used: ORION uses advanced heuristic algorithms, including constrained optimization and machine learning, to process data from over 250 million address points.
- Data Inputs: Package information, customer preferences, real-time traffic data, and driver-specific factors.
Results:
- Fuel Savings: Saved approximately 10 million gallons of fuel annually.
- Cost Reduction: Achieved estimated annual cost savings of $300-$400 million.
- Environmental Impact: Reduced carbon emissions by 100,000 metric tons per year.
Lessons Learned:
- Importance of Driver Collaboration: Involving drivers in the development and implementation process enhanced adoption and effectiveness.
- Continuous Improvement: The system is continually updated with new data to improve performance.
Case Study 2: Daimler's Autonomous Trucks
Overview: Daimler's Freightliner Inspiration Truck was the first licensed autonomous commercial truck, featuring Level 3 autonomy.
Challenges:
- Addressing driver fatigue and related accidents.
- Improving operational efficiency in long-haul trucking.
AI Implementation:
- Technologies Used: Highway Pilot system using radar sensors, stereo cameras, and advanced algorithms for environment recognition and vehicle control.
- Capabilities: Autonomous driving on highways, with driver intervention required for complex situations.
Results:
- Safety Improvements: Potential to reduce accidents caused by human error, which accounts for the majority of trucking accidents.
- Fuel Efficiency: Optimized driving patterns led to fuel savings of up to 5%.
Lessons Learned:
- Regulatory Engagement: Early and proactive engagement with regulators facilitated licensing and testing.
- Public Perception Management: Transparent communication strategies helped build public trust.
Case Study 3: Convoy's Digital Freight Network
Overview: Convoy is a digital freight network that uses AI to optimize freight matching and reduce empty miles.
Challenges:
- High percentage of empty miles in the industry (approximately 35%).
- Inefficient load matching leading to wasted resources.
AI Implementation:
- Algorithms Used: Machine learning models analyze data on shipment details, carrier preferences, historical performance, and real-time location.
- Features: Automated load offers, dynamic pricing, and optimized scheduling.
Results:
- Reduction in Empty Miles: Achieved a 45% reduction in empty miles for participating carriers.
- Increased Carrier Earnings: Improved load utilization led to higher revenue per mile.
- Environmental Impact: Reduced carbon emissions by optimizing route efficiency.
Lessons Learned:
- Data Integration: Success relied on integrating data from various sources, including ELDs and telematics systems.
- Scalability: The platform's ability to scale rapidly was crucial to its effectiveness.
Comprehensive Implementation Roadmap
Assessing AI Readiness
- Data Audit: Evaluate the quantity, quality, and accessibility of data across operations.
- Infrastructure Assessment: Determine if current IT infrastructure can support AI workloads, including data storage and processing capabilities.
- Talent Evaluation: Identify internal expertise in data science, AI, and related fields.
Strategy Development
- Define Objectives: Establish clear, measurable goals for AI implementation (e.g., reduce fuel costs by 10%).
- Prioritize Use Cases: Focus on areas with the highest potential ROI.
- Develop a Roadmap: Create a phased plan with milestones and deliverables.
Timeline and Budgeting
- Phase 1 (0-6 Months):
- Pilot projects in selected areas (e.g., route optimization).
- Initial investment in hardware, software, and training.
- Phase 2 (6-18 Months):
- Expand successful pilots to more routes or fleet segments.
- Begin integrating AI into core business processes.
- Phase 3 (18-36 Months):
- Full-scale deployment across the organization.
- Continuous improvement cycles based on performance data.
Budget Considerations:
- Technology Costs: Hardware upgrades, software licenses, cloud services.
- Human Resources: Hiring data scientists, AI specialists, or partnering with external experts.
- Training and Change Management: Allocating resources for employee training and organizational change initiatives.
Regulatory Landscape Analysis
North America
- United States:
- Federal Motor Carrier Safety Administration (FMCSA): Regulates hours of service, ELD mandates, and safety standards.
- Autonomous Vehicles: Regulatory environment is evolving; the DOT has issued guidelines, but state laws vary.
- Canada:
- Similar regulatory framework to the U.S., with efforts to harmonize cross-border operations.
Europe
- European Union:
- General Data Protection Regulation (GDPR): Strict data privacy laws affecting AI data handling.
- Autonomous Driving Regulations: The EU is working on a cohesive regulatory framework for autonomous vehicles.
Asia-Pacific
- China:
- Aggressive investment in AI and autonomous driving technologies.
- Government support through policies and funding, but with stringent data localization requirements.
- Australia:
- Active trials of autonomous trucks, especially in mining operations.
- Regulatory bodies are developing guidelines for broader use.
Navigating Regulatory Challenges:
- Stay Informed: Regularly monitor regulatory updates and participate in industry forums.
- Compliance Planning: Build compliance considerations into AI implementation plans from the outset.
- Engage with Policymakers: Contribute to policy development through industry associations.
Expert Insights
Chris Spear, President and CEO of the American Trucking Associations:
"AI offers transformative potential for our industry, but we must ensure that we bring our workforce along through training and education."
Dr. Raquel Urtasun, Chief Scientist at Uber Advanced Technologies Group:
"The key to successful autonomous trucking lies in combining cutting-edge AI with rigorous safety validation and collaboration with regulators."
Derek Leathers, CEO of Werner Enterprises:
"Data and AI are becoming as important as the trucks themselves. Companies that leverage these technologies will lead the industry."
John Larkin, Operating Partner at Clarendon Capital:
"AI-driven predictive analytics are revolutionizing maintenance and operations, leading to significant cost savings and efficiency gains."
Rebecca Brewster, President and COO of the American Transportation Research Institute:
"Understanding and addressing the implications of AI on the workforce is critical for the sustainable growth of the trucking industry."
AI's Role in Sustainability
Environmental Benefits
- Emission Reductions: AI-optimized routes and driving behaviors can reduce fuel consumption, lowering CO2 emissions.
- Electric Vehicle Integration: AI can manage electric fleets more efficiently by optimizing charging schedules and routes based on battery life.
Sustainability Goals
- Corporate Social Responsibility (CSR): Aligning AI initiatives with CSR objectives enhances brand image and stakeholder relations.
- Regulatory Compliance: Meeting stricter emission regulations through AI-driven efficiency gains.
Trade-offs and Challenges
- Energy Consumption: AI systems require significant computational power, which consumes energy.
- Lifecycle Impact: Consider the environmental impact of manufacturing and disposing of AI hardware.
Cybersecurity in AI-Driven Trucking
Risks
- Data Breaches: Unauthorized access to sensitive operational data.
- System Hacking: Potential for malicious actors to take control of autonomous vehicles.
- AI Manipulation: Adversarial attacks could trick AI systems into misclassifying data, leading to errors.
Mitigation Strategies
- Robust Encryption: Secure data transmission and storage.
- Regular Security Audits: Proactive identification of vulnerabilities.
- Incident Response Planning: Preparedness for potential cyber incidents.
- AI Security Measures: Implementing AI that can detect and respond to cyber threats in real-time.
Comparative Analysis with Other Industries
Manufacturing
- AI Adoption: Used extensively for predictive maintenance, quality control, and supply chain optimization.
- Lessons for Trucking:
- Automation Integration: Balancing automation with human roles.
- Data Management: Importance of clean, high-quality data.
- Lean Operations: Applying lean principles enhanced by AI analytics.
Healthcare
- AI Adoption: Diagnostics, patient data management, personalized medicine.
- Lessons for Trucking:
- Ethical Considerations: Managing sensitive data responsibly.
- Regulatory Compliance: Navigating complex regulatory environments.
- Patient (Customer) Centricity: Enhancing service delivery through AI.
Actionable Insights for Stakeholders
For C-Suite Executives
- Strategic Vision: Embed AI into the company's long-term strategy.
- Resource Allocation: Invest in technology, talent, and training.
- Risk Management: Develop a comprehensive risk mitigation plan, including cybersecurity and ethical considerations.
- Stakeholder Communication: Keep shareholders, employees, and customers informed about AI initiatives.
For IT Managers
- Infrastructure Development: Ensure scalable and secure IT infrastructure.
- Data Governance: Implement policies for data quality, privacy, and compliance.
- Technology Partnerships: Collaborate with AI vendors and consultants.
- Continuous Learning: Stay updated on AI advancements and cybersecurity threats.
For Fleet Managers
- Operational Integration: Use AI tools for scheduling, routing, and maintenance.
- Performance Metrics: Track KPIs to measure the impact of AI implementations.
- Driver Engagement: Involve drivers in the adoption of AI technologies.
- Safety Focus: Leverage AI to enhance safety protocols and training.
For Drivers
- Skill Development: Embrace training opportunities to work with new technologies.
- Safety Focus: Utilize AI-driven safety features to enhance personal and public safety.
- Feedback Contribution: Provide valuable feedback on AI tools to improve their effectiveness.
- Adaptability: Be open to evolving roles within the industry.
Conclusion
Artificial Intelligence is ushering in a new era for the trucking industry, offering significant opportunities for efficiency, safety, and profitability. By deepening technical understanding, utilizing trucking-specific data, and heeding insights from industry veterans, decision-makers can navigate the complexities of AI adoption. Embracing AI is not just about staying competitive; it's about leading the industry into the future.
References
[1]: McKinsey & Company. (2017). Traveling toward the future: The path for transportation in a post-COVID-19 world. Retrieved from McKinsey
[2]: Frost & Sullivan. (2018). Predictive Maintenance in the Trucking Industry. Retrieved from Frost & Sullivan
[3]: National Highway Traffic Safety Administration. (2015). Critical Reasons for Crashes Investigated in the National Motor Vehicle Crash Causation Survey. Retrieved from NHTSA
[4]: Convoy. (2020). Reducing Empty Miles with Automated Reloads. Retrieved from Convoy Blog
[5]: American Trucking Associations. (2019). ATA Driver Shortage Report. Retrieved from ATA
About Mirage Metrics
At Mirage Metrics, we specialize in delivering cutting-edge AI solutions tailored to the trucking industry. With a team of experts and a deep understanding of industry challenges, we help companies harness the power of AI to transform their operations.
Ready to Lead the Future of Trucking with AI?
Contact us today to embark on your AI journey.
- Book your free consultation with us: https://calendly.com/mehdi-mirage/30min
- Email: solutions@miragemetrics.com
- Website: www.miragemetrics.com
Mirage Metrics—Your Partner in Transformative Innovation
Appendix: AI Readiness Assessment Tool
Note: Access our interactive AI Readiness Assessment Tool on our website to evaluate your organization's preparedness for AI adoption.
Note to the Reader: The case studies presented are based on real-world implementations, with data sourced from credible industry reports and company publications. For the most accurate and up-to-date information, please refer to the original sources provided in the references.